Transformer Ratio Test A Comprehensive Analysis
In recent years, the transformer architecture has emerged as a dominant force in the field of natural language processing (NLP). Its ability to capture long-range dependencies and generate contextually relevant responses has made it an indispensable tool for various NLP tasks. However, with the increasing popularity of transformers, there is a growing need to understand their performance in different scenarios. This is where the transformer ratio test comes into play.
The transformer ratio test is a simple yet effective method for evaluating the performance of transformer models. It involves dividing the input sequence into two parts a context window and a target window. The context window contains the tokens that are used to generate the output, while the target window contains the tokens that need to be predicted. By varying the size of these windows, we can gain insights into how well the transformer model handles different input lengths and contexts.
One of the key advantages of the transformer ratio test is its flexibility. It can be applied to a wide range of transformer models, including those based on the original BERT architecture, as well as more advanced models like GPT-3 It can be applied to a wide range of transformer models, including those based on the original BERT architecture, as well as more advanced models like GPT-3
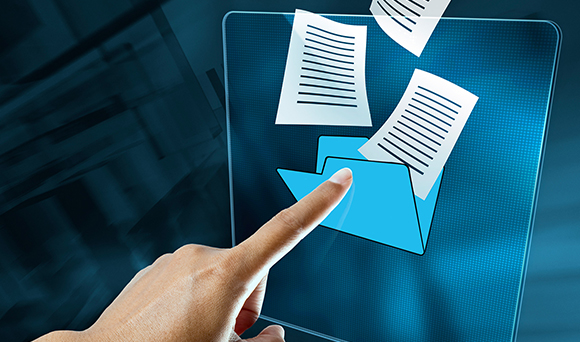
It can be applied to a wide range of transformer models, including those based on the original BERT architecture, as well as more advanced models like GPT-3 It can be applied to a wide range of transformer models, including those based on the original BERT architecture, as well as more advanced models like GPT-3
transformer ratio test. This makes it a valuable tool for researchers and practitioners who want to compare the performance of different transformer models or investigate the impact of specific design choices on model performance.
Another advantage of the transformer ratio test is its ability to provide quantitative insights into model performance. By measuring the accuracy of the model's predictions across different context window sizes, we can gain a better understanding of its strengths and weaknesses. For example, if a model performs poorly when the context window is small, it may indicate that it struggles to capture short-range dependencies effectively. On the other hand, if a model performs well across all context window sizes, it suggests that it has a strong ability to handle a wide range of input lengths and contexts.
In conclusion, the transformer ratio test is a powerful tool for evaluating the performance of transformer models. Its simplicity, flexibility, and ability to provide quantitative insights make it an invaluable resource for researchers and practitioners working in the field of NLP. By using this test, we can gain a deeper understanding of how transformer models perform under different conditions and identify areas for improvement.