The Analysis of Transformer A Game-Changer in NLP
The Transformer, introduced by Vaswani et al. in 2017, has revolutionized the field of natural language processing (NLP) by introducing self-attention mechanisms and eliminating the need for recurrent or convolutional layers. This groundbreaking architecture has since become the backbone of many state-of-the-art models, including BERT, GPT, and T5, among others.
In this article, we will delve into the analysis of the Transformer, exploring its key components, benefits, and limitations. We will also discuss how it has impacted the landscape of NLP and what the future holds for this powerful model.
The Transformer's core innovation lies in its self-attention mechanism, which allows the model to weigh the importance of different words in a sentence based on their relationships with each other. This enables the model to capture long-range dependencies and complex patterns in the data, greatly improving its performance on tasks such as machine translation, sentiment analysis, and question-answering.
One of the key benefits of the Transformer is its ability to handle variable-length sequences, making it highly scalable. Unlike recurrent or convolutional layers, which have fixed input and output sizes, the Transformer can process sequences of arbitrary length, allowing it to handle longer contexts and more complex tasks.
Another advantage of the Transformer is its parallelizability
Another advantage of the Transformer is its parallelizability
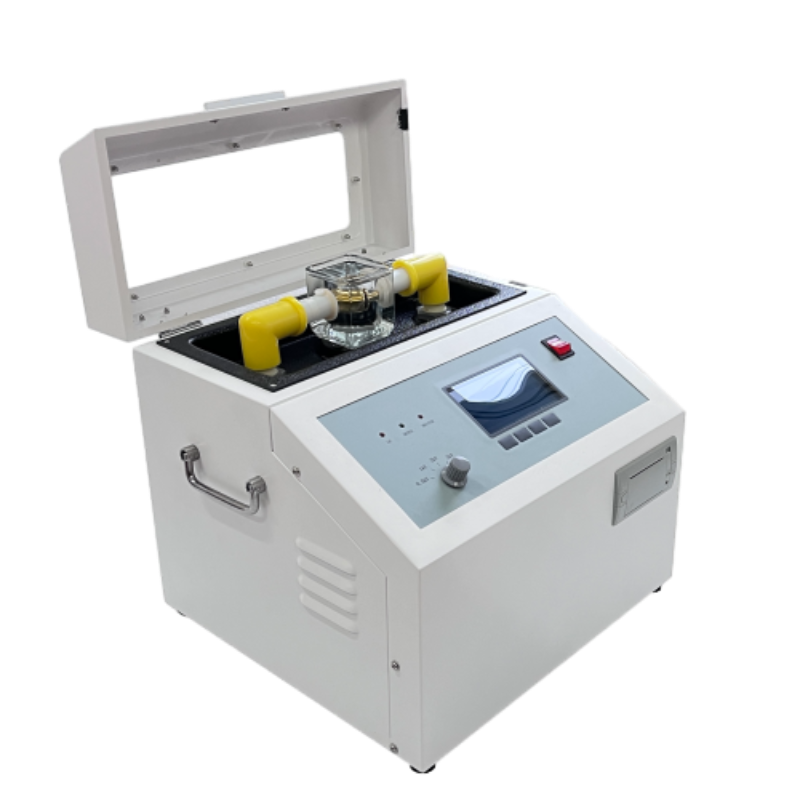
Another advantage of the Transformer is its parallelizability
Another advantage of the Transformer is its parallelizability
analysis of transformer. Since each attention head and feed-forward layer can be computed independently, the entire model can be trained using parallel computing, significantly reducing training time and computational requirements.
Despite its many strengths, the Transformer also has some limitations. One major issue is its high computational cost, which makes it difficult to train on large datasets or deploy on resource-constrained devices. Additionally, the model's sensitivity to hyperparameters and the quality of the pre-training data can also affect its performance.
In conclusion, the Transformer has had a profound impact on the field of NLP, introducing a new paradigm for building powerful and efficient models. Its self-attention mechanism and scalability have made it a go-to architecture for many state-of-the-art models, and its success has inspired researchers to explore new directions in NLP. While there are still challenges to overcome, the Transformer's potential for further advancements in the field is immense.