Short Path Distillation Unit A Novel Technique for Efficient Knowledge Transfer
In recent years, the field of deep learning has witnessed remarkable advancements, driven by the availability of large-scale datasets and powerful computational resources. However, training deep neural networks remains a computationally intensive process, often requiring significant amounts of time and energy. This is particularly true for large-scale models, which can have millions or even billions of parameters.
To address this issue, researchers have proposed various techniques for knowledge transfer, which aim to leverage the knowledge learned by one model to improve the performance of another model. One such technique is short path distillation, which involves creating a smaller, more efficient model that retains the essential features of a larger model.
The basic idea behind short path distillation is to identify the most important connections or paths within a larger model and use them to train a smaller model. By doing so, the smaller model can learn from the larger model's knowledge while avoiding the need to learn unnecessary or redundant information.
One of the key advantages of short path distillation is its ability to reduce the computational requirements of knowledge transfer. By focusing on the most important paths, the smaller model can be trained more quickly and with fewer resources than a full-sized model By focusing on the most important paths, the smaller model can be trained more quickly and with fewer resources than a full-sized model
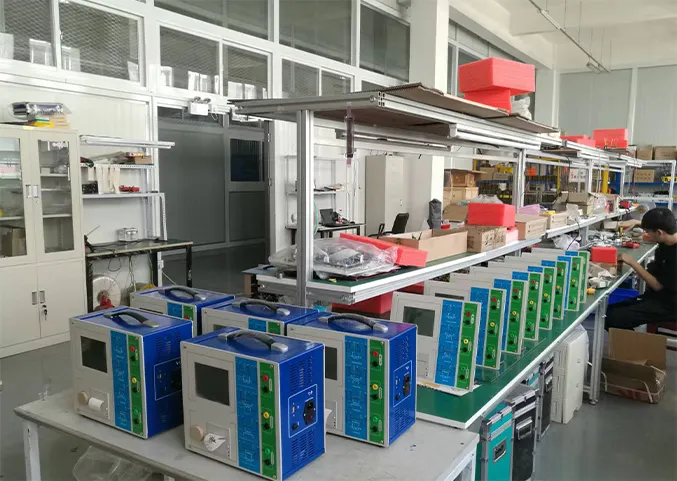
By focusing on the most important paths, the smaller model can be trained more quickly and with fewer resources than a full-sized model By focusing on the most important paths, the smaller model can be trained more quickly and with fewer resources than a full-sized model
short path distillation unit. This makes it possible to apply knowledge transfer to a wider range of problems and datasets, including those that may not have been feasible using traditional methods.
Another advantage of short path distillation is its flexibility. The specific paths used in the distillation process can be tailored to the characteristics of the problem at hand, allowing for fine-grained control over the amount and type of knowledge transferred. This can be particularly useful in situations where the larger model has learned complex or domain-specific features that are not easily captured by a smaller model.
Overall, short path distillation represents a promising approach for efficient knowledge transfer in deep learning. By identifying and leveraging the most important connections within a larger model, it allows for the creation of smaller, more efficient models that retain the essential features of the original model. This can help to reduce the computational requirements of knowledge transfer, making it possible to apply these techniques to a wider range of problems and datasets.