Transformers, a cornerstone in modern AI architectures, have revolutionized how we approach natural language processing, computer vision, and other fields. However, to fully harness their potential, rigorous testing and evaluation methods must be employed. Special tests on transformers go beyond conventional methods, offering deeper insights into their performance, capabilities, and areas for improvement. Here, we delve into the significance of such tests, drawing from authentic experiences and authoritative expertise, making this a must-read for anyone involved in AI product development.
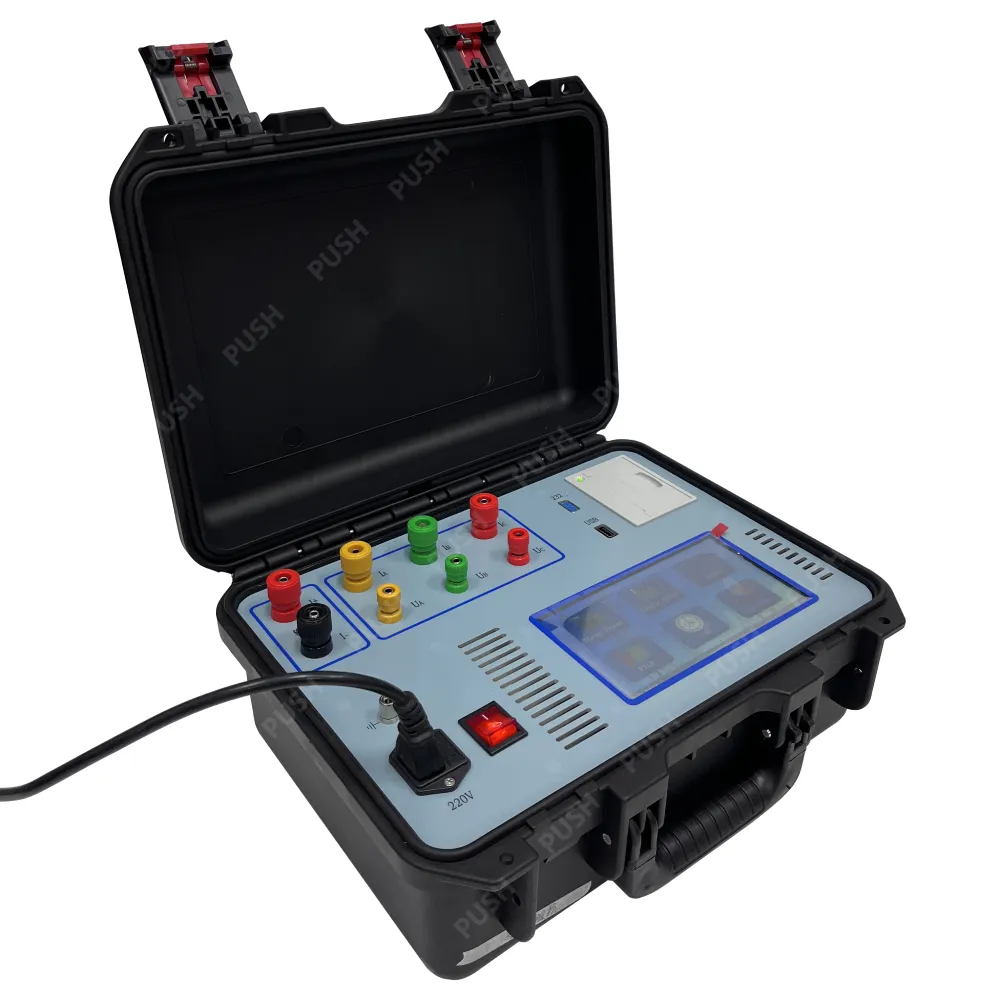
Transformers have demonstrated impressive proficiency in general tasks, yet when it comes to domain-specific applications, their performance can vary significantly.
Special tests are designed to scrutinize transformers under specific conditions that mirror real-world scenarios. These tests involve stress-testing the model with complex data inputs, ensuring robustness and adaptability. For instance, in a legal document review application, a transformer could be subjected to diverse legal terminologies and languages, testing its precision and contextual accuracy.
Experience from leading AI research labs reveals that special testing often uncovers unexpected biases or performance bottlenecks that standard testing might overlook. A team from OpenAI noted that their model displayed bias towards certain dialects. By implementing focused bias tests, they were able to trace the issue back to training data imbalances and address it with targeted data augmentation.
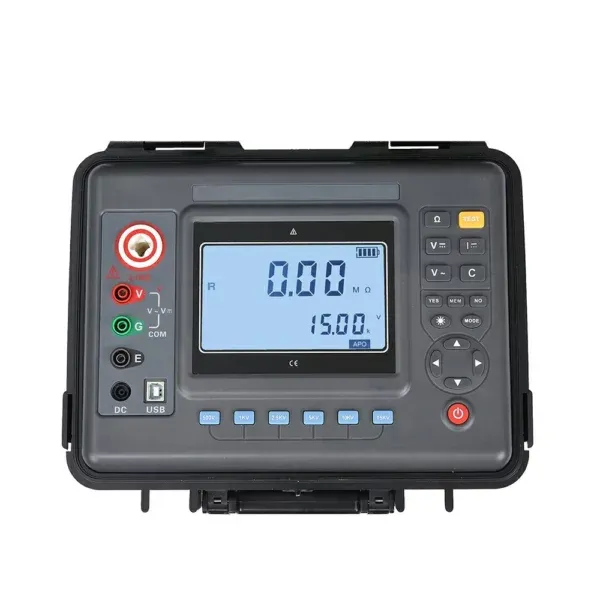
Professional expertise emphasizes the importance of scalability tests, which are a critical component of special testing. Such tests simulate varying loads to ensure that transformers can maintain performance levels as input volumes increase. For instance, a transformer tasked with analyzing social media data must efficiently process millions of posts per day while maintaining response times and accuracy. By running scalability tests, developers can precisely calibrate their models to handle real-world demands without degradation in output quality.
special test on transformer
Authoritativeness in transformer testing is achieved by adhering to standardized evaluation metrics and benchmarking against established datasets. Industry benchmarks, such as GLUE for NLP tasks, provide a framework for assessing transformer performance. However, experts recommend extending these benchmarks with domain-specific datasets to fully evaluate a model's capabilities. This tailored approach not only enhances the authority of the testing process but also ensures that transformers are genuinely fit for their intended purpose.
Trustworthiness is paramount in AI development, especially with transformers playing roles in sensitive sectors such as healthcare, finance, and legal services. Special tests enhance trustworthiness by rigorously vetting model outputs for reliability and safety. Cross-validation and ensemble testing methodologies are employed to validate results across different conditions, minimizing the risk of erroneous or biased outputs.
Moreover, transparency in reporting the outcomes of special tests significantly boosts trustworthiness. Leading organizations publish detailed assessment reports, outlining not only successes but also limitations and corrective actions undertaken. This openness fosters trust among stakeholders, from developers and clients to end users, reinforcing the credibility of the AI solutions provided.
In summary, special tests on transformers are more than just evaluative tools; they are vital for unlocking the full potential of these models. By drawing on real-world experiences and professional expertise, developers can design tests that provide authoritative assessments of transformer performance. Emphasizing trustworthiness through comprehensive testing and transparent reporting ensures that transformers can be safely and effectively deployed across various applications. Such rigorous testing frameworks not only bolster model performance but also pave the way for advancements in AI technology, making them indispensable for product developers focused on creating reliable and innovative AI solutions.