The Transformer Model A Revolution in Natural Language Processing
In the realm of natural language processing (NLP), the advent of transformer models has been nothing short of a paradigm shift. These models have revolutionized the way we interact with and process language data, offering unprecedented accuracy and efficiency.
At the heart of the transformer model is its unique architecture, which diverges significantly from traditional sequence-to-sequence models. Instead of processing data in a linear fashion, the transformer employs a mechanism known as self-attention, allowing it to consider all words in the input sequence simultaneously. This not only speeds up computation but also enables the model to capture complex dependencies between words more effectively.
The transformer's success can be attributed to several factors. Firstly, its ability to parallelize computations across GPUs makes it highly scalable, reducing training time significantly. Secondly, the model's design allows for deeper networks without the vanishing gradient problem, which plagued earlier architectures. This deepening enhances the model's capacity to learn nuanced patterns within language data.
One of the most notable applications of the transformer model is in machine translation. For instance, the Google AI team developed a transformer-based system that outperformed their previous recurrent neural network (RNN) based approach by a wide margin For instance, the Google AI team developed a transformer-based system that outperformed their previous recurrent neural network (RNN) based approach by a wide margin
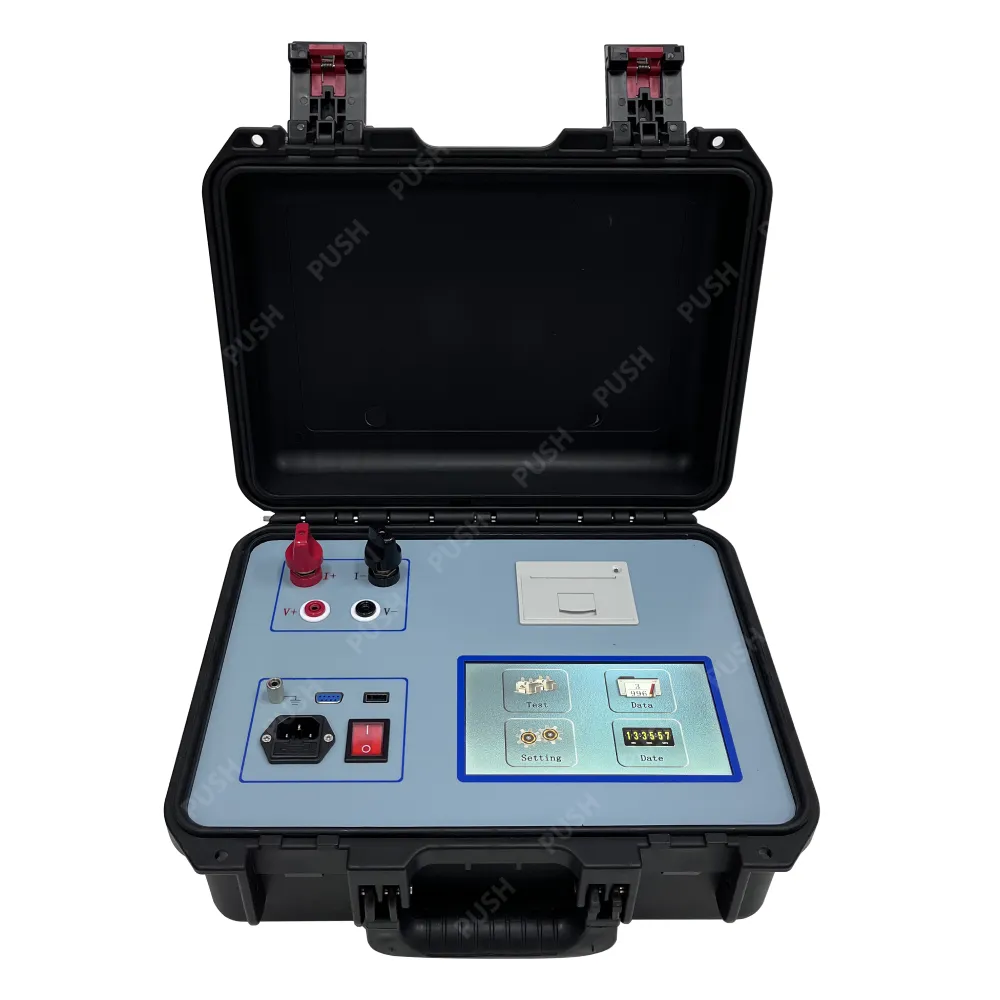
For instance, the Google AI team developed a transformer-based system that outperformed their previous recurrent neural network (RNN) based approach by a wide margin For instance, the Google AI team developed a transformer-based system that outperformed their previous recurrent neural network (RNN) based approach by a wide margin
wrm test of transformer. This improvement was evident not just in translation accuracy but also in the system's ability to handle rare and specialized vocabulary with ease.
Beyond translation, transformers have found use in a myriad of NLP tasks such as text summarization, sentiment analysis, and even generating synthetic text that mimics human writing styles. Their flexibility and adaptability make them an ideal choice for any task involving sequential data.
Despite these advancements, challenges remain. The sheer size of transformer models means they require substantial computational resources, limiting accessibility for researchers and developers with constrained budgets. Additionally, while these models excel at capturing contextual information, they still struggle with understanding the finer nuances of language, such as irony or humor, which are heavily dependent on cultural and situational context.
In conclusion, the wrm test of transformer has undoubtedly propelled NLP forward, showcasing remarkable capabilities in handling complex linguistic data. As research continues, the potential for these models to solve even more intricate language-related problems seems boundless. However, balancing their computational demands with accessibility and further enhancing their understanding of language subtleties remains a focus for future developments in this field.