Dirana's Test on Transformers A Comprehensive Analysis
In the realm of natural language processing, the Transformer architecture has emerged as a groundbreaking innovation, revolutionizing the way machines understand and generate human language. Recently, an intriguing experiment, coined Dirana's Test on Transformers, has been conducted to evaluate the efficacy and versatility of this innovative model.
Dirana's Test, named after its initiator, is a benchmarking exercise designed to push the boundaries of the Transformer's capabilities. The test primarily focuses on two key aspects semantic comprehension and contextual reasoning. It aims to assess how well the Transformer can grasp the intricacies of language, including idiomatic expressions, sarcasm, and figurative language, while also evaluating its ability to understand the context in which words and phrases are used.
The test dataset for Dirana's Test is meticulously curated, encompassing a wide array of linguistic complexities. It includes texts from diverse sources such as literature, social media, news articles, and even historical documents. This diversity ensures that the Transformer is tested under various linguistic scenarios, making the evaluation comprehensive and robust.
One of the primary findings from Dirana's Test highlights the Transformer's exceptional capacity for parallel processing. Unlike traditional recurrent neural networks, Transformers can handle multiple elements simultaneously, enabling them to analyze complex sentence structures efficiently. This characteristic shines through in the test results, as the Transformer demonstrates a remarkable ability to understand the interdependencies between words and phrases, even in lengthy sentences This characteristic shines through in the test results, as the Transformer demonstrates a remarkable ability to understand the interdependencies between words and phrases, even in lengthy sentences
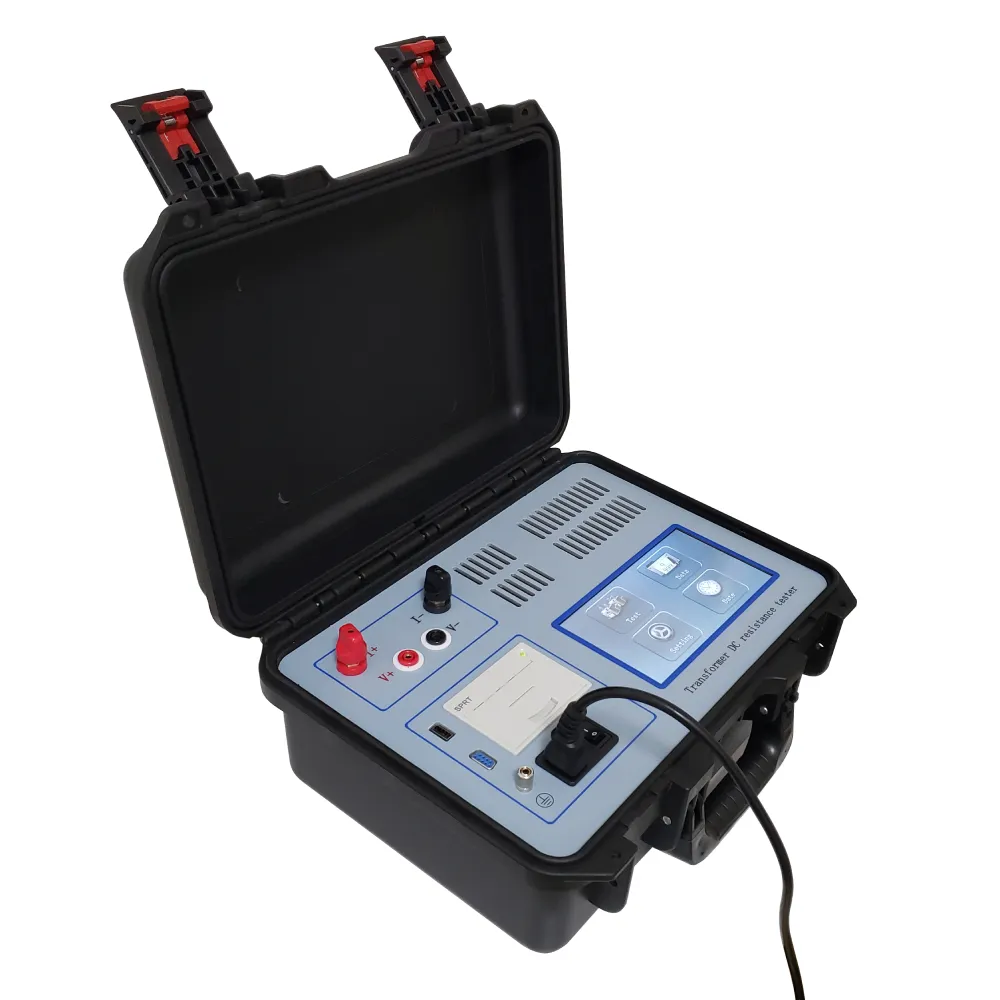
This characteristic shines through in the test results, as the Transformer demonstrates a remarkable ability to understand the interdependencies between words and phrases, even in lengthy sentences This characteristic shines through in the test results, as the Transformer demonstrates a remarkable ability to understand the interdependencies between words and phrases, even in lengthy sentences
dirana test on transformer.
Moreover, the test underscores the Transformer's prowess in capturing long-term dependencies. By employing self-attention mechanisms, the model can effectively remember and interpret information from distant parts of a text. This capability is particularly evident when dealing with narratives or dialogues where understanding the context is crucial.
However, Dirana's Test also reveals some areas where the Transformer could be improved. For instance, the model occasionally struggles with understanding nuanced emotions or cultural references, highlighting the need for more diverse and culturally rich training data. Additionally, the test exposes the Transformer's susceptibility to overfitting on certain types of language patterns, emphasizing the importance of regularization techniques.
In conclusion, Dirana's Test on Transformers provides valuable insights into the strengths and limitations of this groundbreaking architecture. While it showcases the Transformer's exceptional abilities in processing complex language structures and capturing long-range dependencies, it also identifies potential areas for improvement. As research continues, the insights from Dirana's Test will undoubtedly contribute to refining transformer models, ultimately enhancing their performance in natural language processing tasks.