The Impact of PT Transformer on Testing
In recent years, the application of deep learning techniques in natural language processing (NLP) has led to significant advancements in various tasks, such as machine translation, sentiment analysis, and text summarization. One of the most promising approaches in this domain is the Transformer architecture, which has shown remarkable success in handling long-range dependencies and capturing complex patterns in textual data.
However, despite its impressive performance, the Transformer model also presents several challenges when it comes to testing. Traditional testing methods, which rely on manually crafted test cases or rule-based systems, often struggle to effectively evaluate the performance of large-scale language models like the Transformer. This is because these models are highly non-linear and exhibit complex behavior that can be difficult to capture with simple heuristics.
To address these challenges, researchers have proposed a variety of testing techniques specifically designed for evaluating Transformer models. These techniques typically involve generating synthetic test cases that are designed to expose specific weaknesses or limitations of the model. For example, one popular approach is to use adversarial examples, which are carefully crafted inputs that are designed to cause the model to make incorrect predictions For example, one popular approach is to use adversarial examples, which are carefully crafted inputs that are designed to cause the model to make incorrect predictions
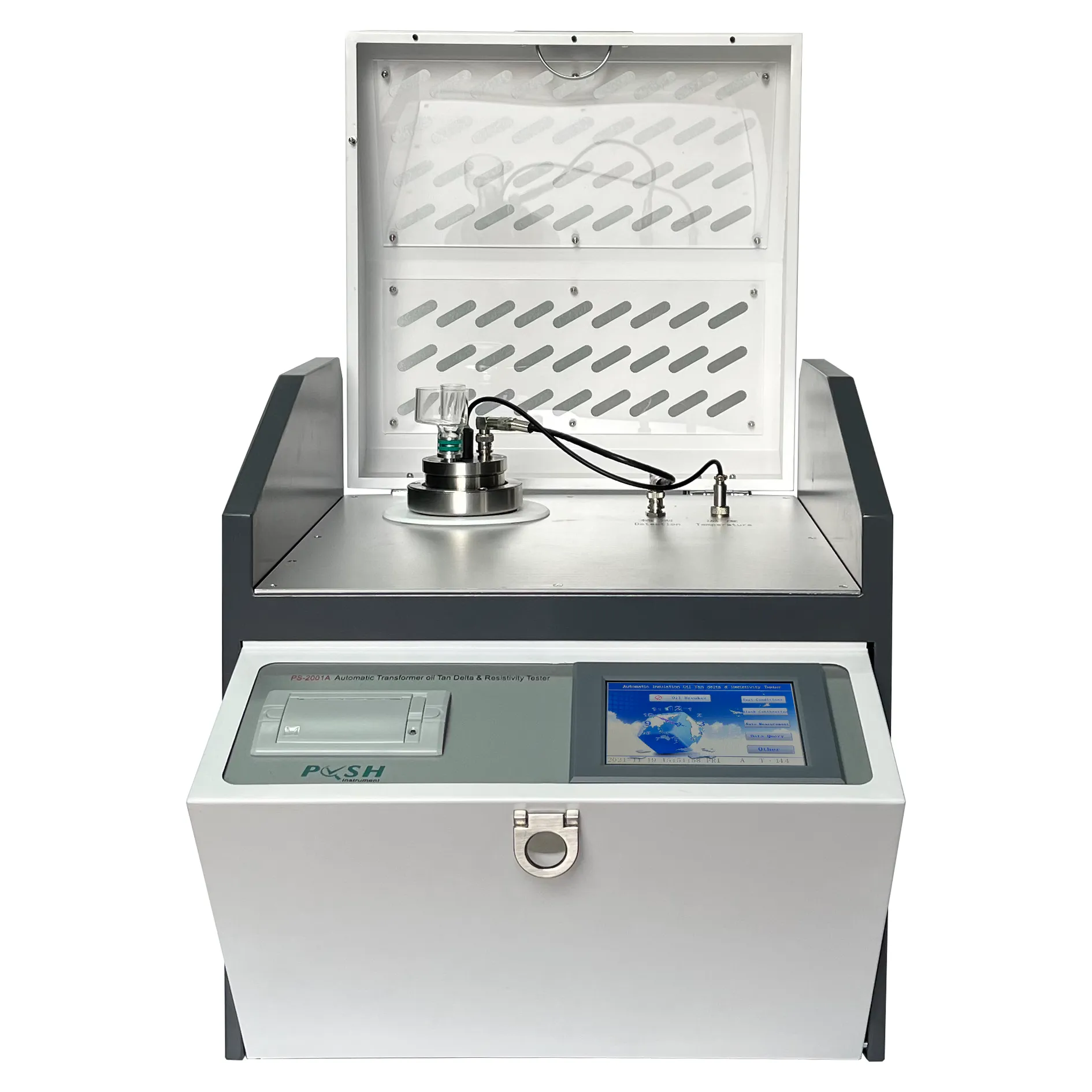
For example, one popular approach is to use adversarial examples, which are carefully crafted inputs that are designed to cause the model to make incorrect predictions For example, one popular approach is to use adversarial examples, which are carefully crafted inputs that are designed to cause the model to make incorrect predictions
pt transformer testing. By analyzing the errors made by the model on these adversarial examples, researchers can gain insights into the model's strengths and weaknesses and develop more effective testing strategies.
Another important aspect of testing Transformer models is the choice of evaluation metrics. While traditional metrics like accuracy and F1 score are still widely used, they may not be sufficient for measuring the performance of complex language models like the Transformer. Instead, researchers are increasingly turning to more sophisticated metrics that take into account factors like fluency, coherence, and semantic correctness.
Overall, the impact of PT Transformer on testing has been significant, leading to the development of new testing techniques and evaluation metrics that are better suited to the unique characteristics of these models. As the use of Transformer-based models continues to grow in NLP applications, it will be essential to continue exploring new testing approaches that can help ensure their reliability and effectiveness in real-world scenarios.