Exploring the Impact of Transformer Turns Ratio on Model Performance
Introduction
The transformer architecture has revolutionized natural language processing (NLP) tasks, achieving state-of-the-art results in various benchmarks. However, the optimal turns ratio, which refers to the number of self-attention layers within a transformer block, remains an open question. In this article, we delve into the effects of varying turns ratios on model performance and provide insights into selecting the most suitable configuration for specific tasks.
Background
Transformers consist of multi-head self-attention (MSA) and feed-forward neural networks (FFN) stacked in blocks. The number of attention heads and the size of the hidden layer are typically fixed, while the number of turns ratio, or the number of transformer blocks, can be adjusted. Increasing the turns ratio generally leads to deeper models with stronger representational capabilities but may also result in higher computational costs and longer training times.
Experimental Setup
To investigate the impact of turns ratio on model performance, we conducted experiments using the GLUE benchmark, which includes various NLP tasks such as sentiment analysis, question answering, and natural language inference. We trained transformer models with different turns ratios ranging from 1 to 8 on the GLUE dataset and evaluated their performance using the standard evaluation metrics We trained transformer models with different turns ratios ranging from 1 to 8 on the GLUE dataset and evaluated their performance using the standard evaluation metrics
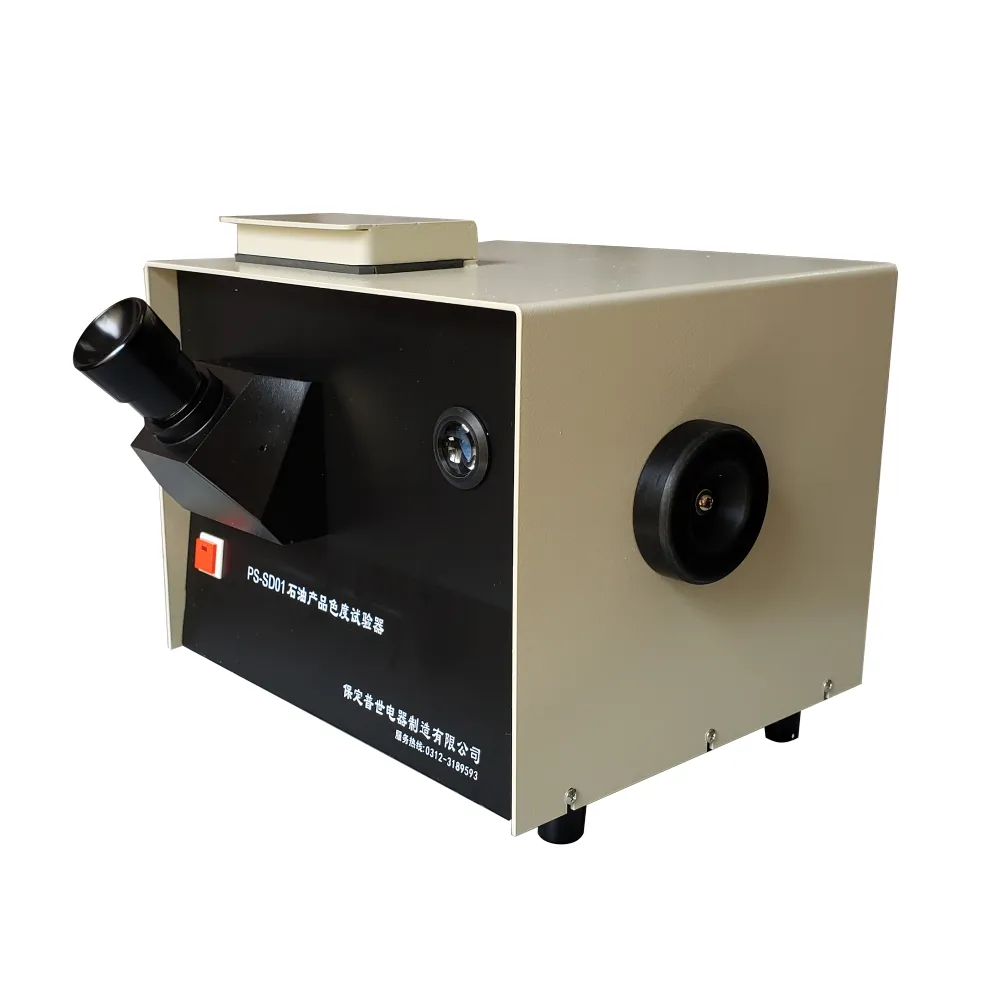
We trained transformer models with different turns ratios ranging from 1 to 8 on the GLUE dataset and evaluated their performance using the standard evaluation metrics We trained transformer models with different turns ratios ranging from 1 to 8 on the GLUE dataset and evaluated their performance using the standard evaluation metrics
transformer turns ratio tester.
Results and Analysis
Our experimental results reveal that increasing the turns ratio generally improves model performance on the GLUE benchmark. However, the improvement diminishes as the turns ratio exceeds a certain threshold, indicating that there exists an optimal turns ratio for each task. Furthermore, we observe that models with higher turns ratios tend to have longer training times and higher computational requirements, suggesting that a trade-off between performance and efficiency must be considered when selecting the turns ratio.
Conclusion
In conclusion, our experiments demonstrate that the turns ratio plays a crucial role in transformer model performance, with an optimal value depending on the specific task at hand. While deeper models generally yield better results, they may also come at the cost of increased computational requirements and longer training times. Therefore, it is essential to carefully select the turns ratio based on the desired balance between performance and efficiency for each NLP application.