The integration of transformers in the cybersecurity landscape is revolutionizing the way we approach Domain Generation Algorithms (DGAs) testing. These algorithms, often employed by cybercriminals to create a plethora of domain names used for malicious command-and-control servers, have become increasingly sophisticated. Understanding the role transformers play in this domain is pivotal for organizations aiming to enhance their cybersecurity infrastructure.
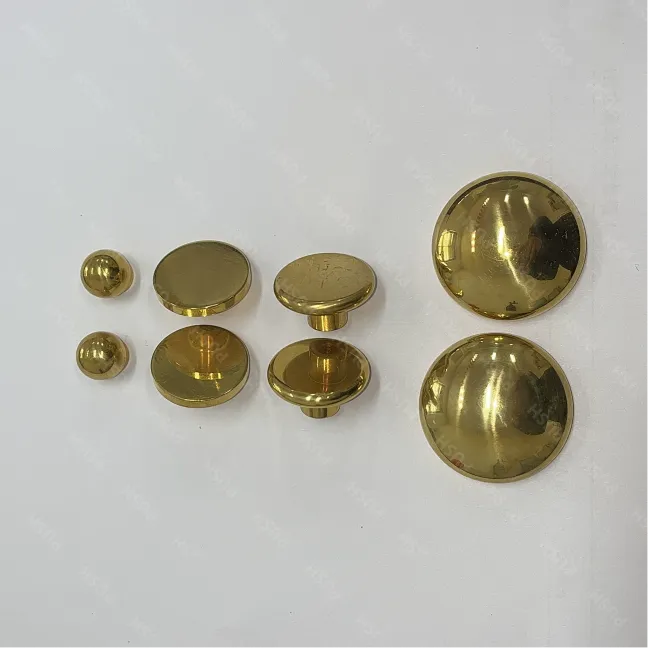
Transformers, a type of machine learning model known for their prowess in natural language processing, have shown remarkable potential in the field of cybersecurity, particularly in detecting and mitigating the threats posed by DGAs. Their ability to analyze context and produce accurate interpretations makes them a valuable asset in understanding the ever-evolving tactics of cyber threats.
The practical application of transformers in DGA detection rests on their capability to sift through massive data sets to identify patterns and anomalies that might escape traditional signature-based systems. By leveraging transformers, security teams can develop robust systems that learn from existing data, adapt to new threats, and anticipate cybercriminal strategies. This dynamic response mechanism ensures that organizations remain one step ahead in the cybersecurity race.
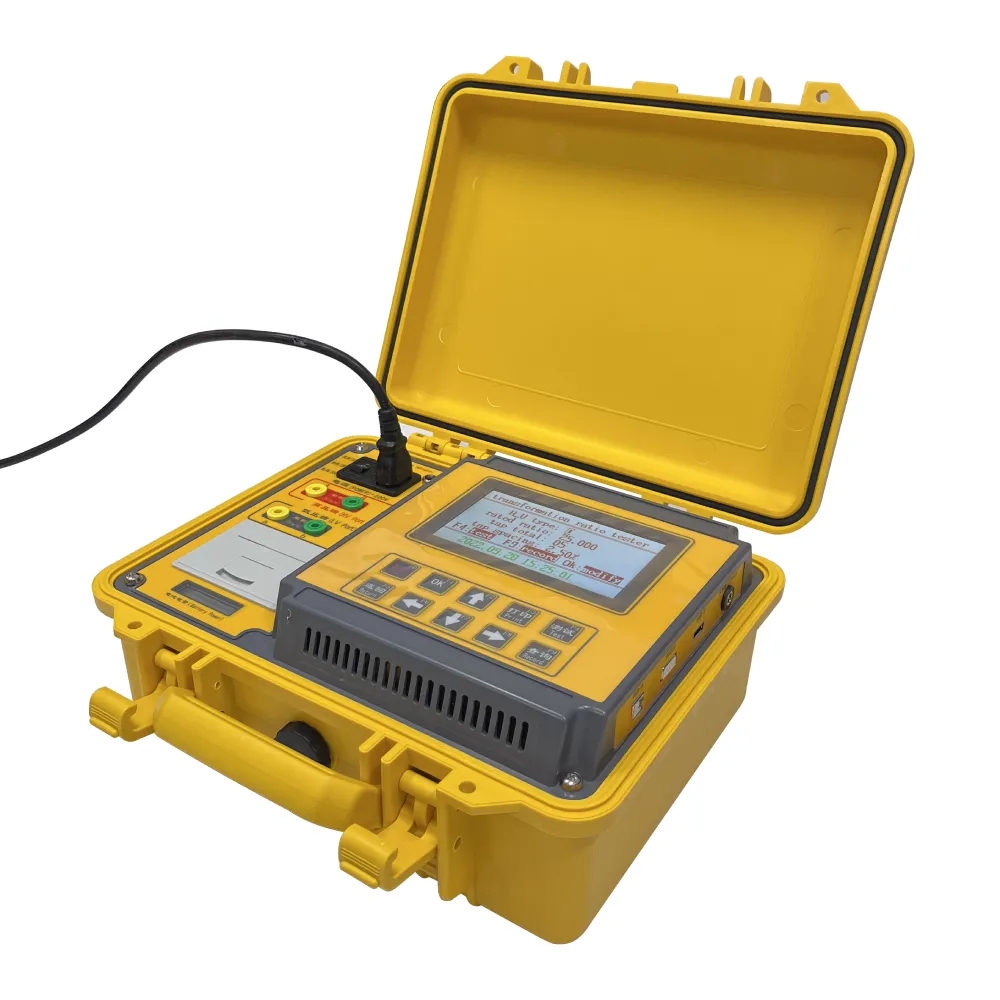
Real-world experiences highlight the effectiveness of transformer models in identifying domains generated by DGAs. Security companies that have implemented transformer-based detection systems report significant reductions in false positives and an increase in the speed and accuracy of threat identification. This is crucial for businesses where time is of the essence in threat neutralization.
transformer dga test
Expertise in deploying transformers for DGA testing involves a deep understanding of both machine learning and cybersecurity principles. Security experts advocate for a comprehensive approach that involves training models with vast datasets containing benign and malicious domain names. This training enables the models to establish a baseline of what typical traffic looks like and to alert on anomalies indicative of DGA activity.
As these models become more prevalent, they contribute significantly to the authoritative body of knowledge in DGA detection. Leading cybersecurity conferences and journals regularly feature research studies and case analyses showcasing the capabilities of transformers. These platforms provide validation and credibility, enhancing trustworthiness in these advanced methods.
Trust is further bolstered when security vendors transparently share the methodologies and datasets used in training their transformer models. Openness in the development process reassures businesses and regulatory bodies of the reliability of these systems. Additionally, partnerships with academic institutions and independent researchers provide third-party validation and peer reviews, strengthening the credibility of transformer-based DGA detection solutions.
The evolution of transformers in the realm of DGA testing signifies a shift towards smarter, more adaptive security solutions. Products integrating these models are on the frontline of cybersecurity, offering robust defenses against ever-changing digital threats. Organizations adopting these innovations not only secure their data but also gain a competitive edge by fostering consumer confidence. In an era where data breaches and cyber threats are commonplace, investing in advanced security measures like transformer DGA testing is not just prudent—it's imperative for sustainable business growth.