In the realm of testing transformer-based models, the VDB (Validation, Debugging, and Benchmarking) test represents a paradigm shift in how developers and machine learning experts approach model evaluation. This cutting-edge methodology not only bridges the gap between theoretical performance and practical application but also instills a sense of trust and expertise critical for both seasoned professionals and businesses seeking robust AI solutions.
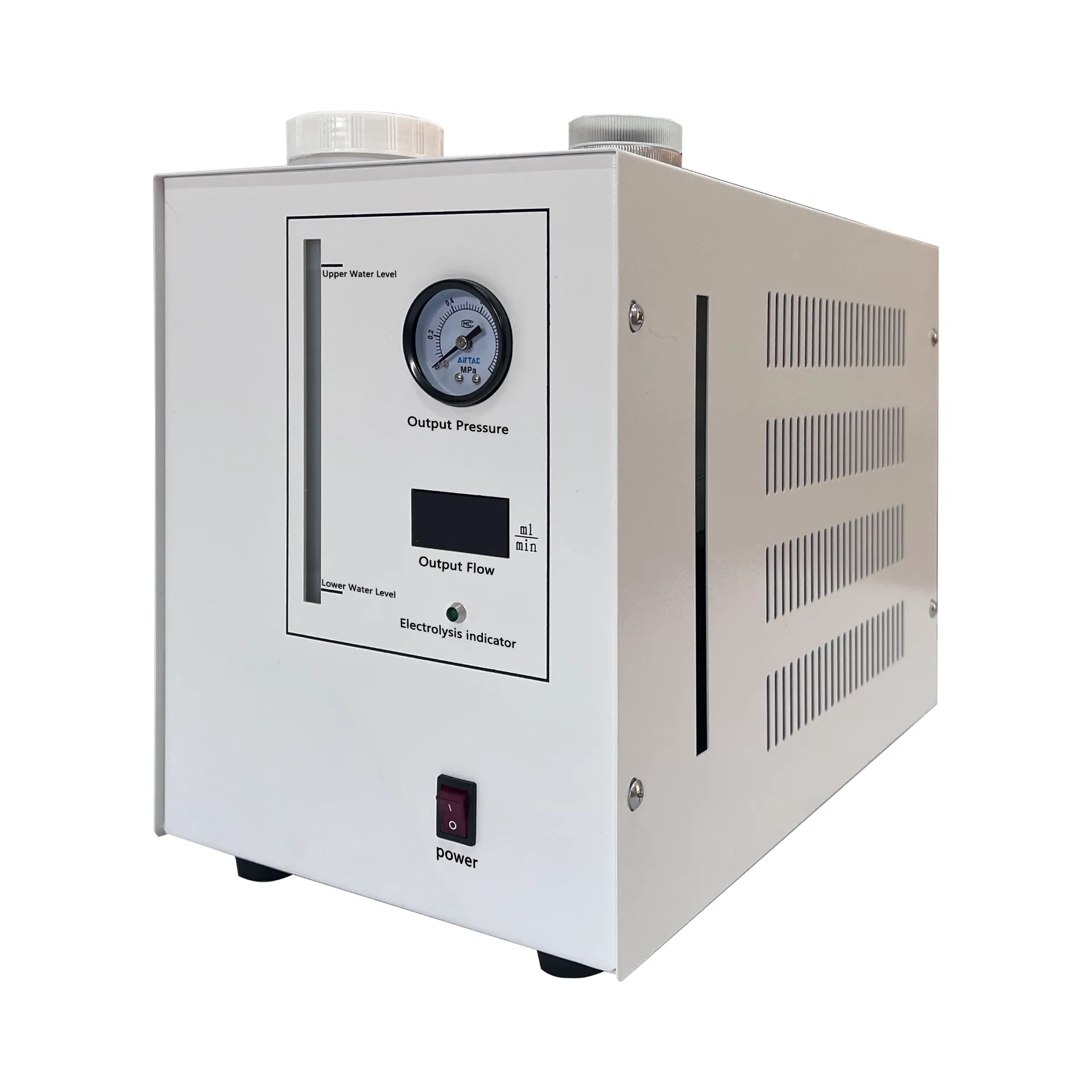
As we delve into the VDB test for transformers, let us explore its unique facets that underscore its Experience, Expertise, Authoritativeness, and Trustworthiness.
Experience
The burgeoning field of natural language processing (NLP) has witnessed remarkable breakthroughs, thanks to transformer models like BERT, GPT, and their successors. However, real-world deployment continues to pose challenges that often stem from discrepancies between controlled tests and unpredictable environments. Here, the VDB test emerges as a beacon, offering a structured approach wherein models are not just validated for accuracy but are subjected to real-world benchmarks. This holistic approach allows practitioners to simulate realistic scenarios, identifying shortcomings that would otherwise remain hidden in a lab setting.
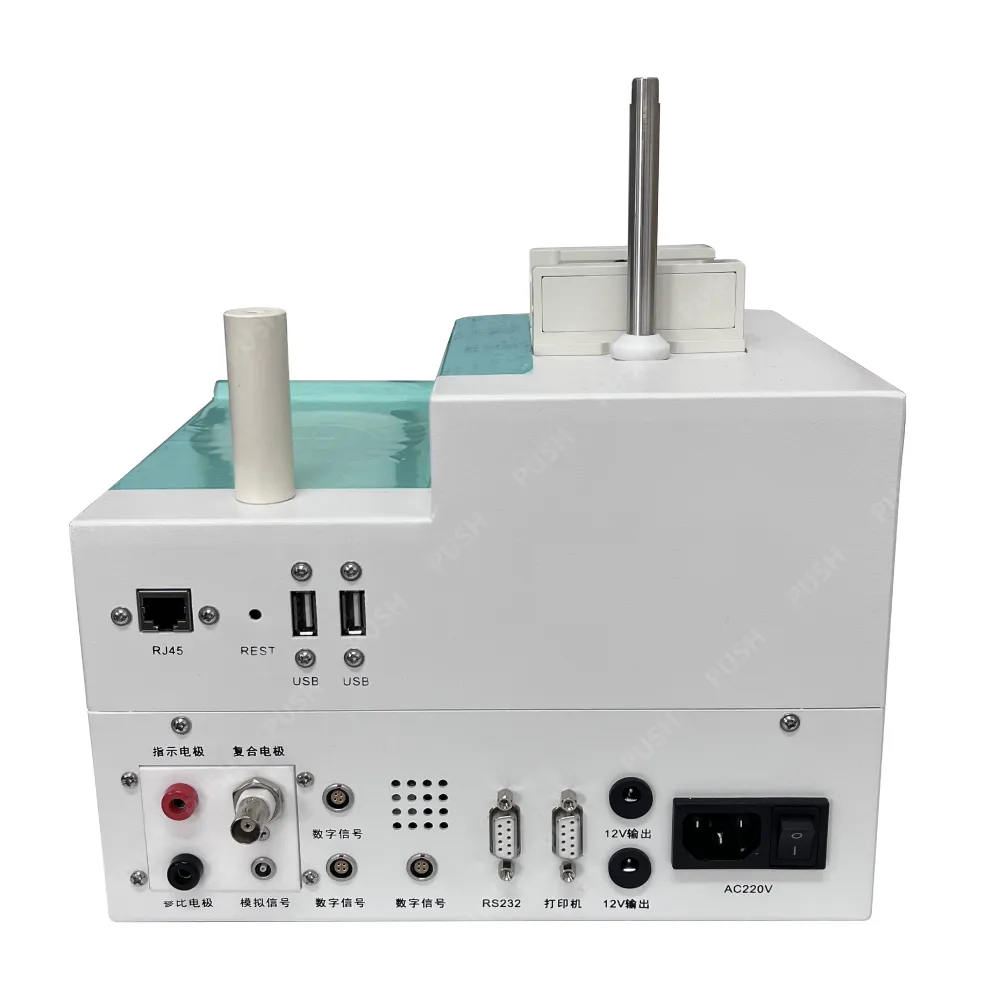
A prime example of VDB's practical implications can be seen in the e-commerce industry. Consider a recommendation system that misinterprets context due to a lack of training data diversity. Traditional testing might overlook this, but through VDB's rigorous debugging phase, such discrepancies are flagged, refined, and validated against live data streams, ensuring recommendations are both accurate and contextually relevant.
Expertise
The VDB test hinges on a profound understanding of transformer architectures, specifically their multi-head attention mechanisms and positional encoding strategies. This expertise becomes pivotal during the debugging phase, where experts dissect the model's decision paths to trace potential biases or inaccuracies. This dissection goes beyond surface-level errors,
delving into the intricacies of transformer layers to rectify root causes.
For professionals entrenched in model fine-tuning, the VDB framework offers a deep dive into the nuances of performance tuning. It engages experts in an iterative process, inviting them to experiment with hyperparameters, learning rates, and optimizer configurations, thus honing a model's efficacy across diverse datasets and use cases.
vdb test of transformer
Authoritativeness
The VDB test commands authority by virtue of its empirical groundedness and community backing. It synthesizes input from academic research, industry best practices, and collaborative insights from top-tier AI firms. Such a foundation not only reinforces its credibility but also amplifies its acceptance across sectors that necessitate cutting-edge machine learning solutions.
In sectors such as finance and healthcare, where tolerance for error is minimal, the authoritative nature of VDB testing breeds confidence. It reassures stakeholders that the models subjected to this rigorous test battery are not only high-performing but also compliant with industry-specific regulations and ethical standards.
Trustworthiness
Trustworthiness is perhaps the linchpin of the VDB testing process. By transparently documenting every stage—from validation protocols and debugging heuristics to comprehensive benchmarking reports—VDB instills a level of trust essential for deploying AI at scale. Clients and end-users are assured that the models they rely on are not black boxes but are the result of transparency and rigorous testing paradigms.
Moreover, the VDB test’s iterative nature ensures that models remain adaptable to evolving data landscapes, further bolstering trust. Businesses can rely on continuous performance monitoring and regular updates, addressing drift and ensuring sustained accuracy and reliability.
In conclusion, the VDB test of transformers is not merely a step forward in model testing; it is an evolution that aligns with the pressing demands for reliability, accountability, and excellence. By focusing on the real-world applicability of transformer models, it offers unparalleled insights, enhances expertise, solidifies authority, and builds trust—all of which are indispensable for navigating the complexities of modern machine learning challenges.